The author is a Director - Finance in Eucloid. For any queries, reach out to us at: contact@eucloid.com
The Definitive Guide to Self-Service Analytics: Benefits, Design, and Emerging Trends
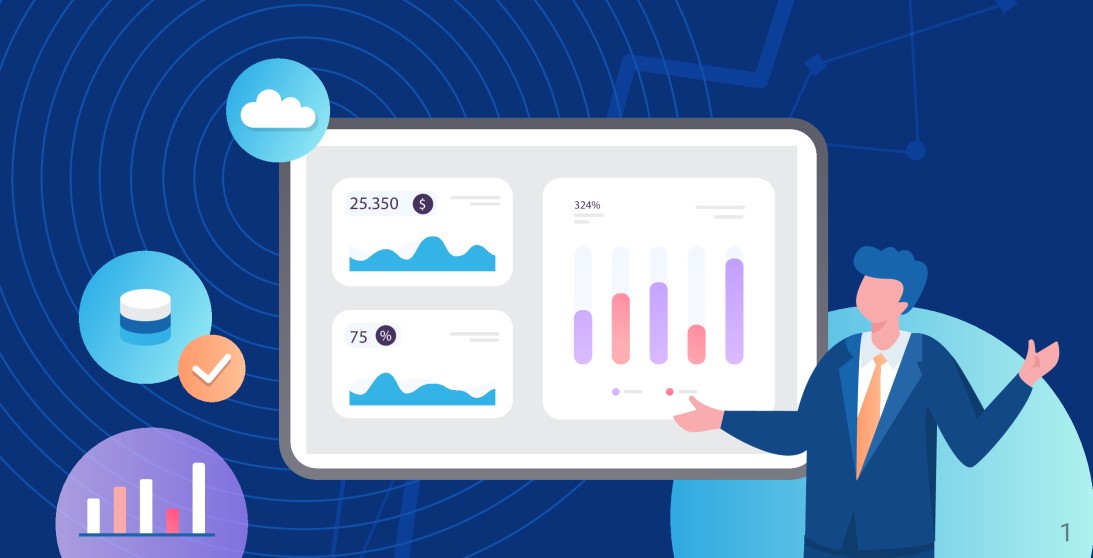
The Current Scenario of Data Requisition
Suppose the CMO of your company needs data of unique visits on the sales page of the company’s mobile app, unique recurrent website traffic on products page, and a 360-degree view of the clients' engagement of a particular region before his urgent client meeting in that geography.
.png)
Overcoming these problems become the building blocks for a self-serve solution.
1. Start with the end: Onboarding and Training the end user
1. Augmented Analytics
Augmented Analytics is an emerging trend within the realm of Self Service Analytics. This cutting-edge approach harnesses the power of artificial intelligence (AI) and machine learning to enhance the analytics process. With Augmented Analytics, tasks such as data cleaning, basic and advanced analysis, and even suggesting insights with commentary are automated, saving valuable time and effort for users. Additionally, the data and trends are intelligently visualized, providing intuitive and actionable insights. By leveraging these AI-driven capabilities, Augmented Analytics empowers users throughout the business hierarchy, from entry-level employees to top-level executives, to confidently make data-driven decisions, fostering a culture of informed decision-making and propelling the organization towards success.
2. Explore data visually
Call it the orthodox way but knowing right visualisation to tell the story is quintessential for making users self serve metrics. Advanced data visualization and exploration will be the next milestone for Self Service Analytics. With compelling charts, graphs, and maps, data exploration will become intuitive and serve as a go-to analysis for a wide range of users, just like a Google search.
3. Data Preparation for business needs
The field of self-service analytics is witnessing yet another emerging trend – the integration of data preparation tailored to specific business needs. This innovative approach enables users to transform raw data into refined and analysis-ready formats. By embedding robust principles of data governance, this process ensures that the data is thoroughly cleansed, sorted, and secured, complying with relevant regulations and privacy standards.
Furthermore, users can access and manipulate the data according to their specific requirements and authorizations, all without the need for IT involvement. This self-service data preparation capability empowers users to swiftly and independently prepare data for analysis, promoting agility and efficiency in decision-making, and accelerating the overall analytics workflow.
4. Embedded Analytics
The future of self-service analytics lies in its integration with various software, applications, and platforms. This means that self-service capabilities will be embedded directly into existing tools such as customer relationship management (CRM), management tools, and business intelligence (BI) platforms.
By eliminating the need for external tools, users can seamlessly access and manipulate data, as well as visualize insights, without having to switch between different applications. This streamlined approach optimizes processes and adds value to products by providing a unified experience.
From a business perspective, this integration saves valuable time, resources, and bandwidth for users, enabling them to focus on deriving meaningful business intelligence.
5. Data Storytelling
The implementation of a Self Service Analytics Solution is poised to revolutionize the art of Data Storytelling. By providing users with intuitive tools and access to vast data repositories, this solution empowers them to unearth profound insights, unveil hidden trends, and uncover intricate relationships within the data.
Armed with this capability, users can craft captivating and persuasive narratives to effectively communicate their findings to the board of stakeholders. With the ability to present compelling and vivid stories, this analytics solution amplifies the impact of data-driven decision-making, fostering a deeper understanding and engagement among stakeholders, ultimately driving organizational growth and success.
Case in Point: From Data to Insights in Marketing
Marketing is an intelligence-driven department that is flooded with data from infinite devices to ever-increasing platforms. Marketing struggles to use such quantities of data and make informed decisions quickly.
The result is an incorrect target audience, disengagement with the customers, an incorrect choice of communication channels, and generic boring messaging to the client.
The opportunities for cross selling and upselling vanish just for the lack of a 360-degree view of the customer.
Self Service Analytics tool steps in to save the day.
The marketing department can run a query or ask in natural language to access the real-time intelligence of the customers. With such insight, they can laser focus their target audience, test the platforms on which the audience interacts and use precisely tailored messaging with impact.
The results of such campaigns can close the open engagements, give the efforts a logical conclusion, and propel marketing efforts in the right direction.
Parting Words
In the tech industry, it’s clear that Self Service Analytics is going to revolutionize the ways of working of the business functions and users that were hitherto challenged by the lack of data expertise. Now, all the sources of data from data lakes to overflowing spreadsheets can be tamed into a Self Service Solution to provide one source of truth for a function and bring agility to the decision making. From the Analytics Solution, the data can be accessed, analysed, visualized, and distributed in a seamless flow. Data Experts can work on sophisticated problems and the Business users can save valuable man hours by fostering a collaborative work environment that thrives on shared knowledge and individual innovation.
The Self Service technology is already on its way to augmented intelligence. The question is when your company will get on this exciting journey and make the most out of your own Self Service Analytics Solution.
Posted on : January 02, 2024
Category : Data Engineering
About the Authors
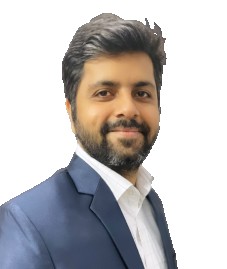
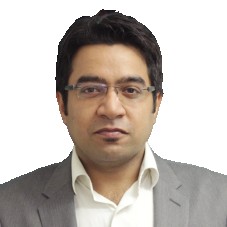
The author is a Director - Delivery in Eucloid. For any queries, reach out to us at: contact@eucloid.com