The author is a Senior Data Engineer in Eucloid. For any queries, reach out to us at: contact@eucloid.com
Composable CDPs with Databricks: A Smarter Way to Know Your Customers
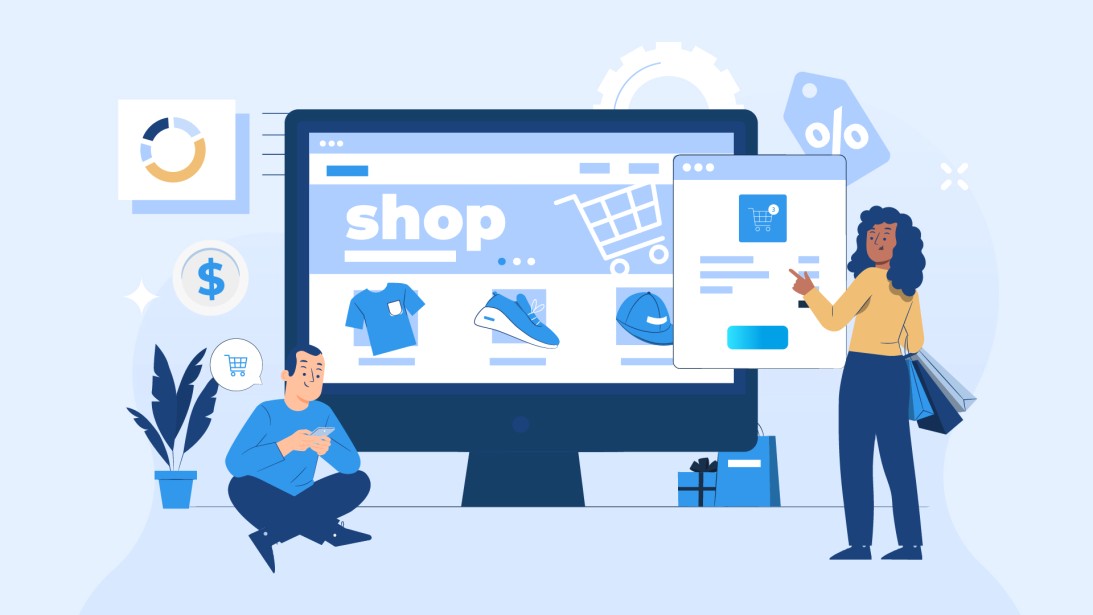
Let’s take an example: a customer browses a product on your website, later sees a related ad on social media, and finally walks into your store to make a purchase. However, your systems treat these as three separate interactions. But is that how it should be? This is when you need to rethink your strategy. And this is also why a Customer Data Platform (CDP) becomes vital.
A CDP acts as a centralized system that gathers, unifies, and integrates customer data across channels such as websites, mobile apps, social media, and offline transactions.
In modern business, a CDP is crucial because it empowers organizations to deliver personalized experiences, improve marketing efficiency, and make data-driven decisions. It breaks down data silos and ensures real-time access to customer insights, helping your business boost engagement, strengthen loyalty, and ultimately drive revenue growth.
Challenges of Packaged Customer Data Platforms (CDPs):
While packaged CDPs offer convenience, they often come with limitations that hinder their effectiveness in dynamic, data-driven environments.
Lack of Flexibility: Most packaged CDPs offer a fixed set of features and integrations. This rigidity can make it difficult to adapt the platform to a company’s unique data architecture or changing business needs.
Vendor Lock-in: Organizations may find themselves dependent on a single vendor’s ecosystem, limiting their ability to innovate or adopt emerging tools.
Limited Customization: Customizing workflows, data models, or processing pipelines can be difficult, especially when the platform does not support deep technical configurations.
Scalability Issues: As data volumes grow and workflows become more complex, some packaged CDPs struggle to scale efficiently, leading to performance bottlenecks.
Integration Barriers: Many CDPs operate as closed systems, making it challenging to integrate with best-in-class tools already in use by marketing, analytics, or data engineering teams.
Composable CDPs: A Smarter Way to Harness Customer Data
Composable CDPs address these limitations by offering a modular, flexible approach built on a company’s existing data infrastructure. Rather than relying on a monolithic system, composable CDPs allow businesses to select and integrate specialized components such as identity resolution, segmentation, activation, and analytics based on their specific requirements. This adaptability not only ensures better alignment with internal tech stacks (e.g. data lakes, warehouses, and real-time pipelines) but also enhances agility, scalability, and control over data governance.
In today’s fast-moving, data-driven market, composable CDPs provide the technological freedom and futureproofing needed to deliver personalized customer experiences without sacrificing performance or innovation.
Key Layers of a Composable CDP
-
Data Collection & Integration Layer
Tools in this layer gather data from various sources and ingest it into your data ecosystem.
Example: Fivetran, Hevo, Snowplow (for website event data)
-
Data Processing Layer
This layer transforms and models raw data into meaningful customer profiles and segments.
Example: Databricks
-
Data Activation Layer
Reverse ETL tools activatecthe final aggregated data from Databricks, creating a sync between CRM platform and Databricks
Example: Hightouch, Census
-
Customer Engagement Layer
This layer powers customer-facing interactions through personalized messaging and campaigns.
Example: MoEngage, CleverTap
Why Databricks for Composable CDP
Databricks is an ideal layer for a composable Customer Data Platform (CDP) because it combines the scalability of a modern data Lakehouse with advanced data engineering, machine learning, and real-time processing capabilities all within a unified platform.
In a composable CDP architecture, flexibility, interoperability, and performance are essential, and Databricks delivers on all three.
-
Unified Lakehouse Architecture: Databricks integrates the best of data lakes and data warehouses, enabling storage and processing of structured and unstructured customer data at scale. This is critical for unifying disparate data sources like web logs, CRM, transactional data, and behavioural signals into a single, analytics-ready format.
-
Open and Composable: Built on open-source technologies like Apache Spark, Delta Lake, and MLflow, Databricks fits seamlessly into modern data stacks. This openness makes it easy to plug into existing tools for identity resolution, audience segmentation, and customer activation without vendor lock-in.
-
Real-Time and Batch Processing: Databricks supports both batch and streaming data through Delta Live Tables and Structured Streaming. This is vital for a composable CDP where real-time personalization and up-to-date customer insights are competitive necessities.
-
Advanced Analytics and AI: With built-in support for machine learning and data science workflows, Databricks enables teams to build predictive models (e.g., churn prediction, lifetime value) and deploy them directly into customer segmentation or personalization flows.
-
Collaboration and Governance: Through features like Unity Catalog and collaborative notebooks, Databricks supports secure, governed, cross-team collaboration—a key requirement for CDP use cases involving marketing, data science, and engineering.
Case study: Transforming Customer Segmentation for an Automotive Brand with Databricks
The segmentation of customers into categories A, B, C is enabled via Mlflow in Databricks. We trained and deployed a classification model and leverage feature store for feature engineering. Moreover, by serving endpoints for LLM models on Databricks, we process the customer sentiments based on call transcripts with customers. The entire model is scheduled by Databricks workflow with file arrival cadence.
Today, we’re seeing more and more use cases where businesses need to move fast, stay flexible, and deeply understand their customers. And composable CDP gives you exactly that: agility, scale, and control. It lets you move beyond one-size-fits-all platforms and build something that fits your business, your tools, and your goals.
At Eucloid, we’ve developed a Composable CDP Accelerator on Databricks, designed to help organizations unify, activate, and maximize the value of their customer data with speed and precision. Get in touch at databricks@eucloid.com to learn how we can help you design and implement a composable CDP that fits your business needs.
Posted on : May 08, 2025
Category : Data Engineering
About the Authors
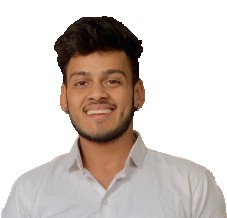